March 26th, 2019
Key Findings
- A machine learning model is shown to be capable of skillfully predicting dissolved oxygen in most regions of the Chesapeake Bay during the peak hypoxia season.
- The vertical density gradient is the dominant driver of recent interannual variability of dissolved oxygen in Chesapeake Bay.
- Skillful forecasts of future dissolved oxygen are possible but will require skillful forecasts of stratification.
- Column-mean water temperature also has an effect on dissolved oxygen consistent with its role in solubility and biological activity.
Andrew C. Ross, Charles A. Stock. Estuarine, Coastal and Shelf Science. DOI: 10.1016/j.ecss.2019.03.007
In parts of many estuaries and other coastal areas, such as the Chesapeake Bay, the concentration of oxygen dissolved in the water regularly drops to a value so low that many species of fish, crabs, and other ecologically and economically important creatures are unable to live. This condition, known as hypoxia, is often driven by warm temperatures and other climate conditions. Subseasonal to seasonal scale forecasting models, including those developed by GFDL, have shown skill at forecasting variations in temperature and other drivers of hypoxia up to several months in advance. Translating these forecasts into skillful forecasts of hypoxia could enable improved management of fisheries, reduce fishing effort, and allow more adaptive management of water quality.
Hypoxia is more likely to occur in warmer weather as the solubility of oxygen is reduced and biological activity is increased in warmer water. Occurrences of hypoxia are also driven by river discharge that is enriched with nitrogen and phosphorus runoff from fertilized land. In addition, as fresh river discharge meets salty ocean water, a vertical density gradient develops, and this density stratification prevents the mixing of oxygen-rich surface water to the bottom. Winds and other weather-scale variability can also influence stratification.
The goal of this research was to determine the predictability of dissolved oxygen and hypoxia in the Chesapeake Bay and to identify the factors that contribute to this predictability. The authors used a machine learning model that takes information about factors known to drive hypoxia in the bay, including stratification, mean temperature, and winds, for a given month and predicts dissolved oxygen concentration during the same month. Compared to previous models, this model has the advantages of resolving the spatial variability of dissolved oxygen and including a larger number of predictors. This model is shown to be capable of skillfully predicting dissolved oxygen in the most regions of the bay during the peak hypoxia season (May to September).
The model results indicate that stratification is the most important variable in the model, and skillful forecasts of future stratification are necessary to use the model to forecast future dissolved oxygen. Stratification is difficult to forecast in advance using a data-driven approach, so future research may focus on the use of numerical ocean models to forecast stratification and dissolved oxygen.
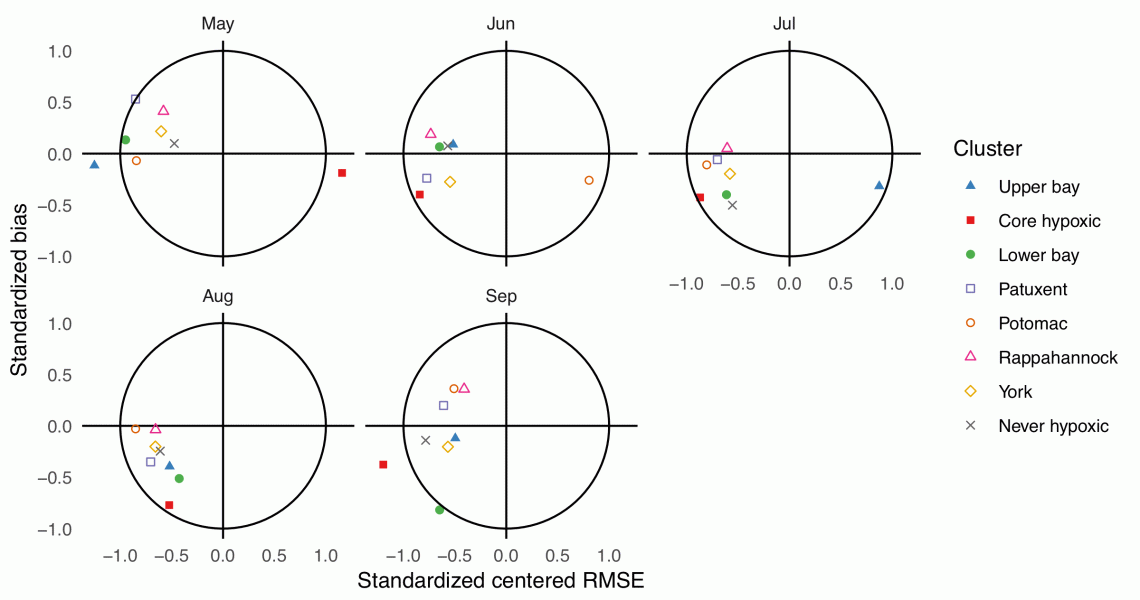