January 22nd, 2018
Key Findings
- A prediction system using global climate models provides skillful prediction of western U.S. snowpack for March, based only on observational data from the preceding July 1st (i.e. the prediction is skillful 8 months in advance)
- This physically based dynamic system outperforms observation-based statistical predictions made on July 1st for March snowpack everywhere except the Southern Sierra Nevada, a region where prediction skill is presently nonexistent
- When negative trends in snowpack are removed, the prediction skill in the maritime mountains (i.e. Washington, Oregon Cascades, Sierra Nevada) is reduced. These regions have the highest hydroclimate variability, particularly in the Sierra Nevada, posing a challenge for any prediction system due to their more chaotic nature.
Sarah B. Kapnick, Xiaosong Yang, Gabriel A. Vecchi, Thomas L. Delworth, Rich Gudgel, Sergey Malyshev, P.C.D. Milly, Elena Shevliakova, Seth Underwood, Steven A. Margulis. Proceedings of the National Academy of Sciences. DOI: 10.1073/pnas.1716760115
The majority of annual precipitation in the Western United States accumulates between October and April, falling as snow in the mountains. As a result, snow accumulation forms mountain snowpack, peaking in early spring and melting into the summer, dominating runoff and influencing lower elevation streamflow. Variations in snowpack influence warm season water supply, wildfire risk, ecology, and industries like agriculture dependent on snow and downstream water availability. Efforts to understand snowpack variability have predominantly been focused on either weekly (weather) or decadal to centennial (climate variability and change) timescales. The authors focused on a timescale in between, demonstrating that a global climate model suite can provide snowpack predictions 8-months in advance. These predictions from climate models outperform statistical methods from observations alone. The results show that seasonal hydroclimate predictions are possible, and highlight areas for future prediction system improvements.
Mountainous regions in the Western U.S. are characterized by strong winter storms, sometimes only a few per year, which produce heavy rains at low elevations and snow in the mountains. During the spring and summer while precipitation is scarce, snowmelt from the mountains feeds rivers, reservoirs, and ecology. Up to 80% of snowmelt runoff is used for agriculture. In years with low precipitation or high temperatures, snowpack is reduced, leading to summer and fall drought conditions.
Intense interest in creating seasonal snow predictions developed after the multi-year drought in California (2012-2015). The high priority need for seasonal snowpack predictions was also reflected in the Weather Research and Forecasting Innovation Act of 2017 (H.R. 353), signed into law in April 2017.
While climate projections provide estimates of snowpack loss by the end of the century and weather forecasts provide predictions of weather conditions out to two weeks, less progress has been made for snow predictions at seasonal timescales (months to 2 years), crucial for regional agricultural decisions (e.g. plant choice and quantity). Seasonal predictions with climate models first took the form of El Niño predictions 3 decades ago, with hydroclimate predictions emerging more recently. While the field has been focused on single-season predictions (3 months or less), this work shows that we are now poised to advance predictions beyond this timeframe. Utilizing observations, climate indices, and a suite of global climate models, the authors demonstrate the feasibility of seasonal snowpack predictions and quantify the limits of predictive skill 8 months in advance.
This physically-based dynamic system outperforms observation-based statistical predictions made on 1 July for March snowpack everywhere except the Southern Sierra Nevada, a region where prediction skill is nonexistent for every predictor presently tested. Additionally, in the absence of externally forced negative trends in snowpack, narrow maritime mountain ranges with high hydroclimate variability pose a challenge for seasonal prediction in our present system. Natural snow pack variability may inherently be unpredictable at this time scale. Or, our current prediction system is inadequate to produce snowpack predictions in this region, and models should be further developed specifically to improve regional skill in these elongated coastal ranges.
This work highlights present prediction system successes and gives cause for optimism for developing seasonal predictions for societal needs. The authors have identified potential improvements for seasonal predictions for water resource needs.
The initialization methodology (how the models are started on July 1) has not been optimized for higher resolution atmosphere-land models and focuses on ocean conditions. This may introduce errors as the model spins up, inhibiting prediction skill. This is akin to the difference between getting on the highway at the speed of high-speed traffic versus trying to go from 0 to 60 mph, to merge. Some years will be fine, but in others you will get a crash from the mismatch.
Further improvements to global climate models (e.g. mountain precipitation, gravity waves, snowpack hydrology, aerosols, spatial resolution) may improve prediction skill. Additionally, observations are used in the prediction system for starting up the models via initialization and for verification of the forecasts. Observational products designed for these purposes are crucial.
Data sets used for prediction and verification
Data Type | Description |
---|---|
Prediction: Global Climate Models | 3 NOAA global climate models with different atmosphere-land resolutions (200, 50, 25 km) |
Prediction: Observed July 1st climate index values | Pacific Decadal Oscillation (PDO), Pacific North American pattern (PNA), and El Niño Southern Oscillation (ENSO) index values |
Verification: Observed western U.S. snowpack | Snow point observations were used to develop a new snow product from 1981-2016 across the entire western U.S. |
Verification: California snowpack reanalysis | Blended remote-sensing and point station based product for Sierra Nevada snowpack 1985-2016 |
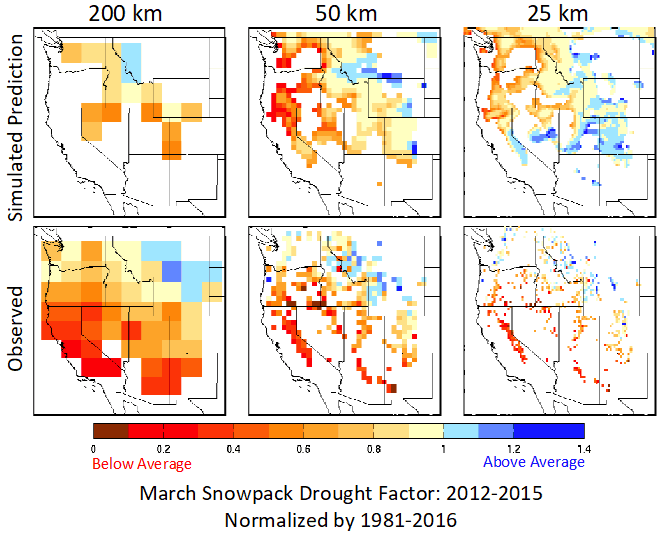
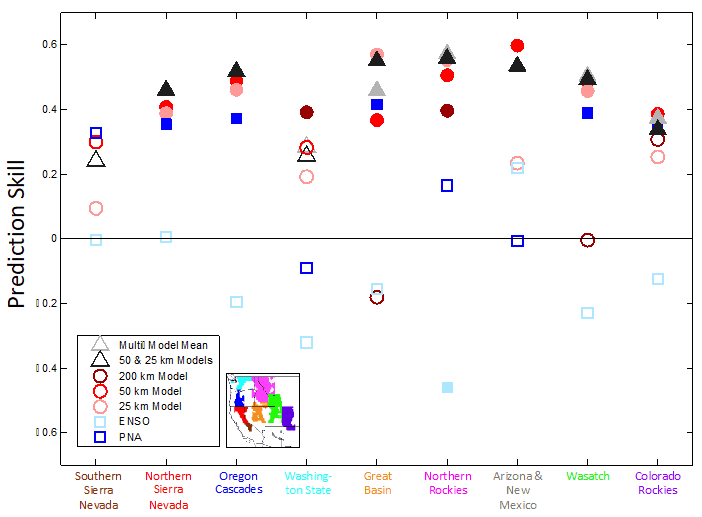