March 6th, 2015
Key Findings
- The new high-resolution FLOR model shows skillful seasonal predictions of near-surface air temperature and precipitation over land.
- The skill in seasonal precipitation over land arises primarily from the El Niño-Souther Oscillation (ENSO) phenomenon.
- Both a multi-decadal change (in part, from external forcing) component and a spatially heterogeneous ENSO-related component contribute to the seasonal prediction skill of near surface air temperature over land.
- Reconstructing predictions by filtering out unpredictable components in the model improves seasonal skill compared to raw predictions from the model.
Liwei Jia, X. Yang, G.A. Vecchi, R.G. Gudgel, T.L. Delworth, A. Rosati, W.F. Stern, A.T. Wittenberg, L. Krishnamurthy, S. Zhang, R. Msadek, S. Kapnick, S. Underwood, F. Zeng, W. G. Anderson, V. Balaji and K. Dixon. Journal of Climate. DOI: 10.1175/JCLI-D-14-00112.1.
Summary
Skillful seasonal predictions of surface temperature and precipitation over land are in demand, due to their importance to ecosystems and sectors such as agriculture, energy, transportation. This study demonstrates skillful seasonal prediction of near-surface air temperature and precipitation over land using a new high-resolution climate model developed at GFDL, called FLOR. The study also diagnoses the sources of the prediction skill.
This study shows that the use of refined statistical analysis and a high-resolution dynamical model leads to significant skill in seasonal predictions of near-surface air temperature and precipitation over land. Output from predictions with FLOR is being made available to NOAA’s National Weather Service (and the world) through the North American Multi-Model Ensemble for Seasonal Prediction (NMME).
The authors employ a statistical optimization approach to identify the most predictable components of seasonal temperature and precipitation over land. The two most predictable components of near-surface air temperature are characterized by a spatially homogeneous component that is mostly due to changes in external radiative forcing in both boreal winter and summer, and a spatially heterogeneous ENSO-related pattern in boreal winter. The most predictable components of precipitation in boreal winter and summer are also ENSO-related. These predictable components of temperature and precipitation show significant correlation skill for all leads from 0 to 9 months. Importantly, the reconstructed predictions based only on the leading few predictable components from the model show considerably better skill relative to observations than raw model predictions.
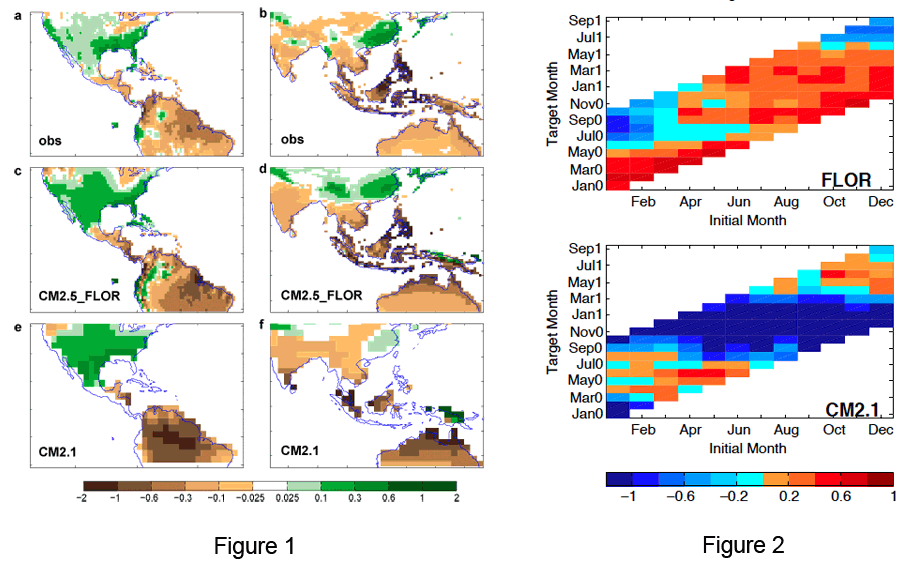